Improving Accuracy
Bauer Assistant Professor Receives Best Paper Award at Hong Kong Conference for Fintech, AI and Big Data in Business
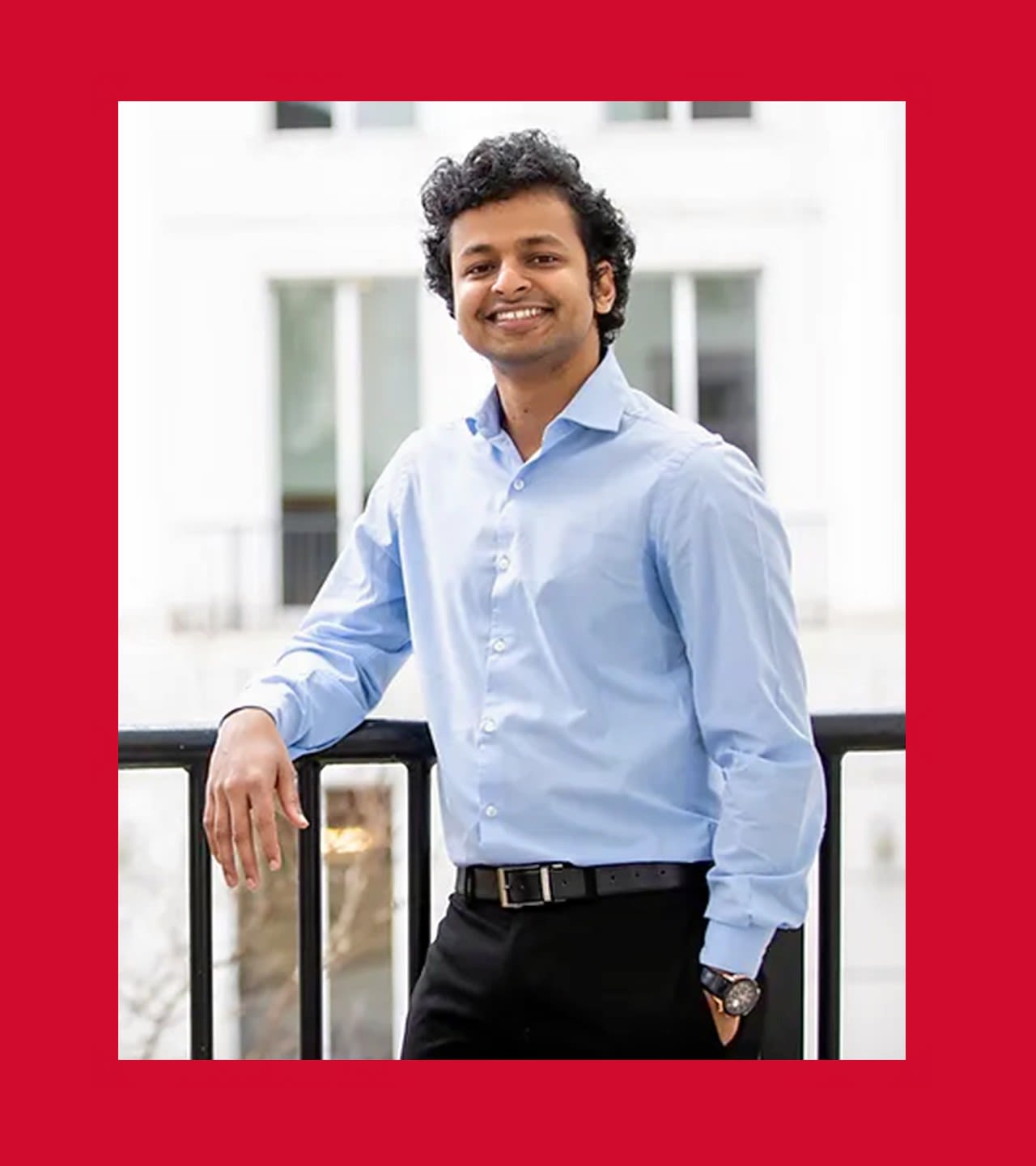
Investment portfolios based on stock return forecasts made by machine learning models have created a buzz in recent years. Their outperformance of standard investment strategies portends a potential revolution in the field of finance.
Improving the accuracy of those predictions further has huge implications, and a C. T. Bauer College of Business finance researcher recently was honored at an international conference for his development of a mathematical model with the potential to do just that.
Assistant Professor Rohit Allena received the Best Paper Award at the Hong Kong Conference for Fintech, AI, and Big Data in Business for his research, “Confident Risk Premiums and Investments using Machine Learning Uncertainties,” which he intends to resubmit for publication in The Review of Financial Studies.
Allena, who joined Bauer in 2021, said he noticed that the precision (i.e., confidence intervals) of the stock return forecasts made by machine learning models had largely been ignored in broader discussions of machine learning investment models. Forecasts with wide confidence intervals, for instance, are likely to have high forecast errors, Allena said. Forecasts that are more precisely measured by AI models are likely to have low forecast errors.
“Exploiting this intuition, I make two contributions in my research. First, I explicitly show how to estimate confidence intervals for return forecasts that are based on a wide range of machine learning models such as LASSO, Ridge, Elastic Net, and Neural Networks,” Allena said. “Second, based on the precision of return forecasts, I introduce improved investment portfolios, which I refer to as “Confident-high-low” trading strategies. These investment portfolios strategically take long (short) positions exclusively on stocks that are more confidently forecasted to deliver higher (lower) returns in the next period.
“The practical implications of the Confident-high-low strategies can be huge,” Allena said. “Most hedge funds and asset managers typically construct investment portfolios based solely on return forecasts made by various models. These strategies are typically known as high-low strategies. I argue that Confident-high-low strategies that incorporate the information from the precision of return forecasts, in addition to the information from the return forecasts, will deliver significantly superior investment returns relative to the high-low strategies.”
He added: “The Confident-high-low portfolios outperform because they include only stocks with relatively more precise return forecasts, ignoring investments that are prone to high forecast errors. To my knowledge, this is the first paper in finance to estimate confidence intervals of stock return forecasts that are based on machine Learning models. This exercise is very challenging given the sophisticated mathematical structure of machine learning models.”
“The insight that the information from the confidence intervals is useful for constructing improved investment portfolios is also novel to the literature. Most researchers and practitioners typically focus solely on return forecasts, ignoring their confidence intervals, while constructing investment portfolios,” he said.
Allena came to Bauer College after earning a doctoral degree at Emory University. One of his dissertation essays won a Cubist Systemic Strategies award for outstanding research at the Western Finance Association annual meeting in 2020. His research focuses on asset pricing and market-microstructure, with an emphasis on the econometrics of machine learning inferences and Bayesian inferences.